In the digital thread and digital twin vision, testing data and CAE data are integrated into PLM (product lifecycle management) to exploit historical product-related information. This is linked to data from real-time physical asset operations, thanks to IoT. The digital thread enables different departments to access and collaborate on data, facilitating effective communication, version control, traceability and operations on a digital twin of the physical product or process.
Data-driven predictions recycle data and give it a new life thanks to predictive engineering models executable by any engineer. AI can create accessible predictive tools and collaboration opportunities for such diverse departments as R&D, product design, manufacturing, quality assurance, cost estimation, product and program management and sales engineering.
The key to the democratization and acceleration of engineering is an AI branch called deep learning.
Continuous improvement in engineering prediction speed
Deep learning can outperform humans and human-design algorithms in terms of speed and accuracy of engineering predictions.
By human-designed algorithm, we mean software developed and designed by humans based on their expertise and knowledge in a particular field. An example in engineering is CAE software that incorporates physics principles into the analysis of engineering systems such as battery crashworthiness or entire vehicle aerodynamics.
In predicting the air wake of a car based on its geometry and operating conditions or reproducing a crash box test, CAE speed performance compared to physical cycles of prototyping and testing is particularly advantageous.
Deep learning is a further step building on CAE and testing, proving even more extraordinary by using data to enhance the CAE predictive speed by orders of magnitude to obtain results in seconds instead of hours. The role of testing is in no way ignored. In a data-driven approach, all data is useful and can be weighted/blended into a single AI predictive model.
Example battery housing case study
Engineers can estimate the likelihood of damage to the battery cells during a collision by measuring energy absorption and deformation during the crash test or a simulation. Data generated by product designers (CAD) and application engineers (CAE) can be used to train deep learning efficiently. The advantage of the trained deep learning model is not only having CAE run faster (one second instead of one hour) for this type of test. Now, all company engineers (from cost estimators to R&D) can access AI without specific AI skills and visualize the predictions on a new design.
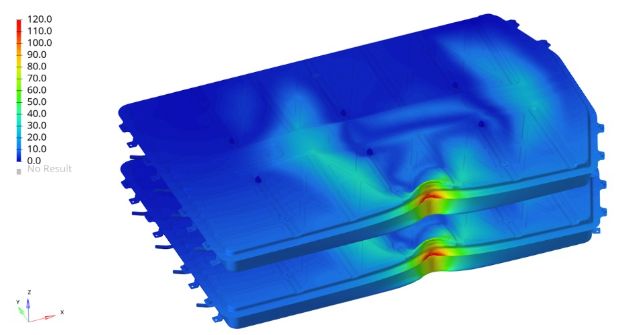
Are AI predictions reliable?
Rather than on one sample case, the comparison between AI and CAE or testing is made on many instances (tens or hundreds of CAE simulations or laboratory experiments). The figure shows a statistical measure of confidence on the y-axis called R² (R²=1 being equal to perfection compared to reference data, and R²=0 meaning no correlation). This is shown in an engineering result such as the maximum force predicted during the crash test. If users choose an acceptance threshold not to trust 10% of the predictions, based on this indicator, the R² score would be 0.95 (very high) on the 90% of trusted predictions.
Deep learning with data fusion: Incorporating testing data and CAE data
We have seen two different sources of data for deep learning: CAE and CAD data within a digital thread and testing data that can be coupled with a parametric or CAD geometry description. Can we integrate this data, resulting in next-generation predictive analytics tools for the automotive industry to create a comprehensive and enhanced understanding?
Data fusion combining multiple data sources, is the answer. Data fusion involves integrating different types of data. AI architectures like convolutional neural networks (CNNs) or recurrent neural networks (RNNs) can effectively capture spatial and temporal dependencies, enabling the model to learn from both data types.
Making AI available to all engineers
Following testing and simulation, AI will not replace them but rather exploit their data to create value by shortening design cycles and making engineering predictions available to more departments in automotive companies, thanks to AI-based engineering apps. In analogy, AI-based engineering apps promote cross-department collaboration in automotive companies between such diverse profiles as testing engineers, simulation engineers, product designers, program managers and cost estimators.
The possible role of AI in EV testing
AI-powered algorithms can analyze sensor data collected from EVs during real-world testing. These algorithms can detect patterns and anomalies in the data, providing valuable insights into vehicle performance, safety and reliability.
Another potential role for AI in EV testing is in autonomous driving. AI algorithms can be trained to simulate complex driving scenarios and evaluate the performance of autonomous systems.
Simulation of EVs is an area of recent and impactful progress for AI. AI algorithms can generate accurate and detailed virtual environments to simulate various driving conditions, weather patterns and road scenarios. This allows manufacturers to conduct extensive testing without needing physical prototypes.
During the design phase, AI can also optimize EV components or full vehicles, with vehicle aerodynamics or battery crashworthiness, by analyzing vast amounts of data generated by testing and CAE.
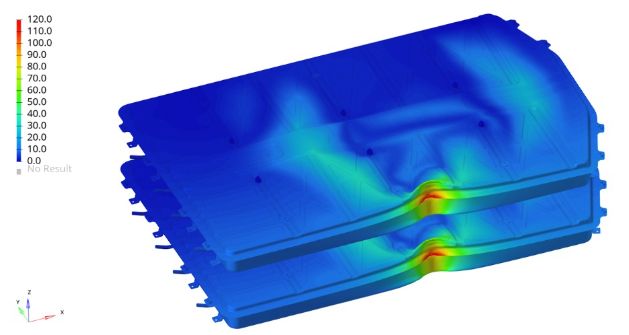
The availability of testing, CAE and CAD data in the same digital thread, such as a PLM system, facilitates AI learning from engineering data. There has been a competition between testing and CAE simulation, where testing provides more realistic data while simulation makes it much less expensive and faster to produce them. This competition was already reconciled within the digital thread and PLM.
Testing and simulation have become collaborative by fusion of data and creating next-generation predictive analytics tools for the automotive industry. AI promises to unite the best of the two worlds, while automotive engineers will benefit from this union.